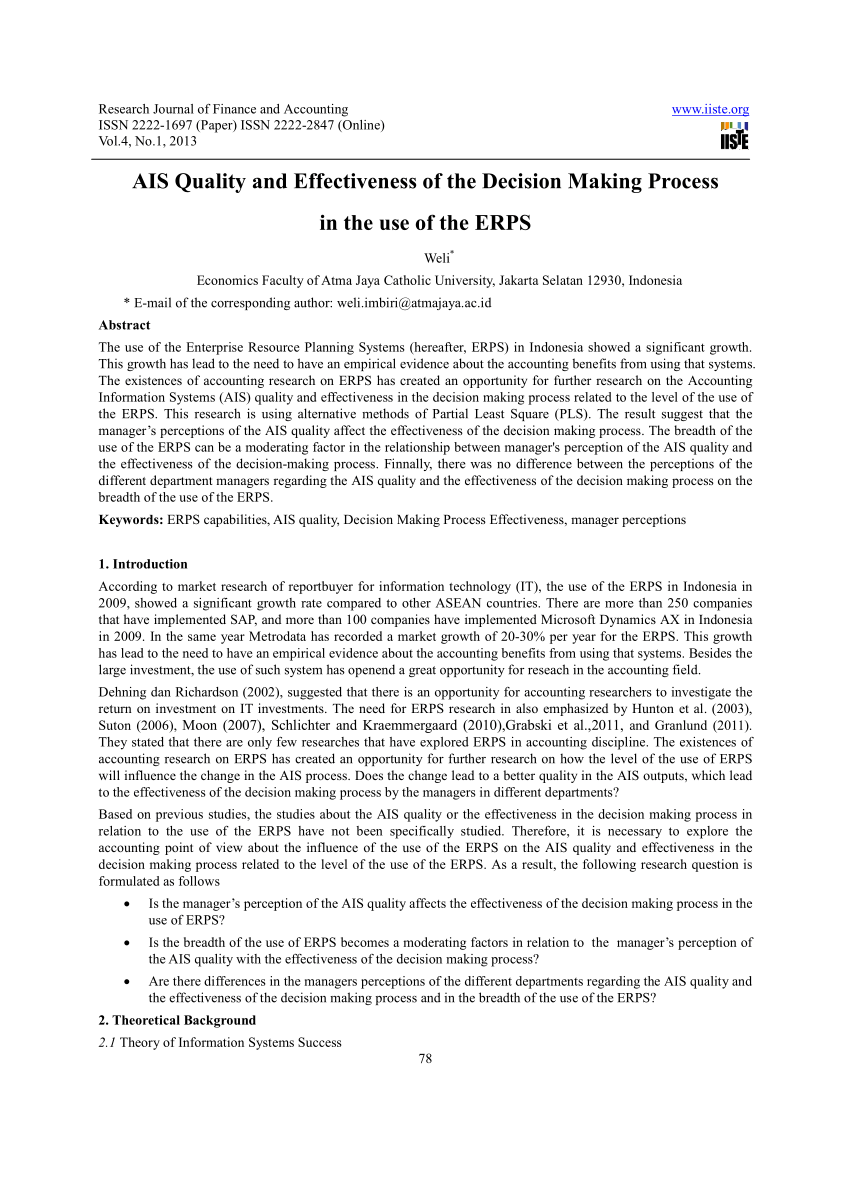
An information system (IS) is a formal, sociotechnical, organizational system designed to collect, process, store, and distribute information. From a sociotechnical perspective, information systems are composed by four components: task, people, structure (or roles), and technology. Information systems can be defined as an integration of components for collection, storage and processing of data Get 24⁄7 customer support help when you place a homework help service order with us. We will guide you on how to place your essay help, proofreading and editing your draft – fixing the grammar, spelling, or formatting of your paper easily and cheaply We can handle your term paper, dissertation, a research proposal, or an essay on any topic. We are aware of all the challenges faced by students when tackling class assignments. You can have an assignment that is too complicated or an assignment that needs to
Business Topics & Research Titles about Business
In artificial intelligencean expert system is a computer system emulating the decision-making ability of a human expert. The knowledge base represents facts and rules.
The inference engine applies the rules to the known facts to deduce new facts. Inference engines can also include explanation and debugging abilities.
Soon after the dawn of modern computers in the late s — early s, researchers started realizing the immense potential these machines had for modern society. In particular, making these machines capable of making important decisions the way humans do.
Thus, in the late s, right after the information age had fully arrived, researchers started experimenting with the prospect of using computer technology to emulate human decision-making. For example, biomedical researchers started creating computer-aided systems for diagnostic applications in medicine research paper on expert system for financial planning biology.
However, researchers had realized that there were significant limitations when using traditional methods such as flow-charts [12] [13] statistical pattern-matching, [14] or probability theory.
This previous situation gradually led to the development of expert systems, which used knowledge-based approaches. These expert systems in medicine were the MYCIN expert system, [17] the INTERNIST-I expert system [18] and later, in the middle of the s, the CADUCEUS. Expert systems were formally introduced around [20] by the Stanford Heuristic Programming Project led by Edward Feigenbaumwho is sometimes termed the "father of expert systems"; other key early contributors were Bruce Buchanan and Randall Davis.
The Stanford researchers tried to identify domains where expertise was highly valued and complex, such as diagnosing infectious diseases Mycin and identifying unknown organic molecules Dendral, research paper on expert system for financial planning. The idea that "intelligent systems derive their power from the knowledge they possess rather than from the specific formalisms and inference schemes they use" [21] — as Feigenbaum said — was at the time a significant step forward, since the past research had been focused on heuristic computational methods, culminating in attempts to develop very general-purpose problem solvers foremostly the conjunct work of Allen Newell and Herbert Simon.
Research on expert systems was also active in France. While in the US the focus tended to be on rules-based systems, first on systems hard coded on top of LISP programming environments and then on expert system shells developed by vendors such as Intellicorpin France research focused more on research paper on expert system for financial planning developed in Prolog, research paper on expert system for financial planning.
The advantage of expert system shells was that they were somewhat easier for nonprogrammers to use. The advantage of Prolog environments was that they were not focused only on if-then rules; Prolog environments provided a much better realization of a complete first order logic environment. In the s, expert systems proliferated. Universities offered expert system courses and two thirds of the Fortune companies applied the technology in daily business activities.
Inthe first IBM PCwith the PC DOS operating system, was introduced. The imbalance between the high affordability of the relatively powerful chips in the PC, compared to the much more expensive cost of processing power in the mainframes that dominated the corporate IT world at the time, created a new type of architecture for corporate computing, termed the client—server model. This model also enabled business units to bypass corporate IT departments and directly build their own applications.
As a result, client research paper on expert system for financial planning had a tremendous impact on the expert systems market. Expert systems were already outliers in much of the business world, requiring new skills that many IT departments did not have and were not eager to develop.
They were a natural fit for new PC-based shells that promised to put application development into the hands of end users and experts. Until then, the main development environment for expert systems had been high end Lisp machines from XeroxSymbolicsand Texas Instruments.
With the rise of the PC and client server computing, vendors such as Intellicorp and Inference Corporation shifted their priorities to developing PC based tools. Also, new vendors, often financed by venture capital such as Aion Corporation, Neuron DataExsys, and many others [27] [28]started appearing regularly. The first expert system to be used in a design capacity for a large-scale product was the SID Synthesis of Integral Design software program, developed in SID expanded the rules and generated software logic synthesis routines many times the size of the rules themselves.
Surprisingly, the combination of these rules resulted in an overall design that exceeded the capabilities of the experts themselves, and in many cases out-performed the human counterparts. While some rules contradicted others, research paper on expert system for financial planning, top-level control parameters for speed and area provided the tie-breaker.
The program was highly controversial, but used nevertheless due to project budget constraints. It was terminated by logic designers after the VAX project completion.
During the years before the middle of the s, the expectations of what expert systems can accomplish in many fields tended to be extremely optimistic. At the beginning of these early studies, researchers were hoping to develop entirely automatic i. The expectations of people of what computers can do were frequently too idealistic. This situation radically changed after Richard M. His findings describe what computers can do and what they cannot do.
Many of the computational problems related to this type of expert systems have certain pragmatic limitations. These findings laid down the groundwork that led to the next developments in the field. In the s and beyond, the term expert system and the idea of a standalone AI system mostly dropped from the IT lexicon.
There are two interpretations of this. One is that "expert systems failed": the IT world moved on because expert systems did not deliver on their over hyped promise. The limitations of the previous type of expert systems have urged researchers to develop new types of approaches. They have developed more efficient, flexible, and powerful approaches in order to simulate the human decision-making process. Some of the approaches that researchers have developed are based on new methods of artificial intelligence AIand in particular in machine learning and data mining approaches with a feedback mechanism.
Related is the discussion on the disadvantages section. Modern systems can incorporate new knowledge more easily and thus update themselves easily. Such systems can generalize from existing knowledge better and deal with vast amounts of complex data.
Related is the subject of big data here. Sometimes these type of expert systems are called "intelligent systems. An expert system is an example of a knowledge-based system. Expert systems were the first commercial systems to use a knowledge-based architecture.
A knowledge-based system is essentially composed of two sub-systems: the knowledge base and the inference engine. The knowledge base represents facts about the world. In early expert systems research paper on expert system for financial planning as Mycin and Dendral, these facts were represented mainly as flat assertions about variables. In later expert systems developed with commercial shells, research paper on expert system for financial planning, the knowledge base took on more structure and used concepts from object-oriented programming.
The world was represented as classes, subclasses, and instances and assertions were replaced by values of object instances. The rules worked by querying and asserting values of the objects. The inference engine is an automated reasoning system that evaluates the current state of the knowledge-base, applies relevant rules, and then asserts new knowledge into the knowledge base. The inference engine may also include abilities for explanation, so that it can explain to a user the chain of reasoning used to arrive at a particular conclusion research paper on expert system for financial planning tracing back over the firing of rules that resulted in the assertion.
There are mainly two modes for an inference engine: forward chaining and backward chaining. The different approaches are dictated by research paper on expert system for financial planning the inference engine is being driven by the antecedent left hand side or the consequent right hand side of the rule.
In forward chaining an antecedent fires and asserts the consequent. For example, consider the following rule:.
A simple example of forward chaining would be to assert Man Socrates to the system and then trigger the inference engine. It would match R1 and assert Mortal Socrates into the knowledge base. Backward chaining is a bit less straight forward. In backward chaining the system looks at possible conclusions and works backward to see if they might be true. So if the system was trying to determine if Mortal Socrates is true it would find R1 and query the knowledge base to see if Man Socrates is true.
One of the early innovations of expert systems shells was to integrate inference engines with a user interface. This could be especially powerful with backward chaining. If the system needs to know a particular fact but does not, then research paper on expert system for financial planning can simply generate an input screen and ask the user if the information is known.
So in this example, it could use R1 to ask the user if Socrates was a Man and then use that new information accordingly. The use of rules to explicitly represent knowledge also enabled explanation abilities. In the simple example above if the system had used R1 to assert that Socrates was Mortal and a user wished to understand why Socrates was mortal they could query the system and the system would look back at the rules which fired to cause the assertion and present those rules to the user as an explanation.
In English, if the user asked "Why is Socrates Mortal? A significant area for research was the generation of explanations from the knowledge base in natural English rather than simply by showing the more formal but less intuitive rules. As expert systems evolved, research paper on expert system for financial planning, many new techniques were incorporated into various types of inference engines.
The goal of knowledge-based systems is to make the critical information required for the system to work explicit rather than implicit. With an expert system the goal was to specify the rules in a format that was intuitive and easily understood, reviewed, and even edited by domain experts rather than IT experts.
The benefits of this explicit knowledge representation were rapid development and ease of maintenance. Ease of maintenance is the most obvious benefit. This was achieved in two ways.
First, by removing the need to write conventional code, many of the normal problems that can be caused by even small changes to a system could be avoided with expert systems. Essentially, the logical flow of the program at least at the highest level was simply a given for the system, simply invoke the inference engine. This also was a reason for the second benefit: rapid prototyping. With an expert system shell it was possible to enter a few rules and have a prototype developed in days rather than the months or year typically associated with complex IT projects.
A claim for expert system shells that was often made was that they removed the need for trained programmers and that experts could develop systems themselves. In reality, this was seldom if ever true. While the rules for an expert system were more comprehensible than typical computer code, they still had a formal syntax where a misplaced comma or other character could cause havoc as with any other computer language.
Also, as expert systems moved from prototypes in the lab to deployment in the business world, issues of integration and maintenance became far more critical. Inevitably demands to integrate with, and take advantage of, large legacy databases and systems arose. To accomplish this, integration required the same skills as any other type of system. The most common disadvantage cited for expert systems in the academic literature is the knowledge acquisition problem. Obtaining the time of domain experts for any software application is always difficult, but for expert systems it was especially difficult because the experts were by definition highly valued and in constant demand by the organization.
As a result of this problem, a great deal of research in the later years of expert systems was focused on tools for knowledge acquisition, to help automate the process of designing, debugging, and maintaining rules defined by experts.
Podcast #16 How to Become Your Own Bank Featuring Financial Planning Expert Mark Willis
, time: 41:12Prospective Employees | Job Opportunities | Careers at UW | Human Resources
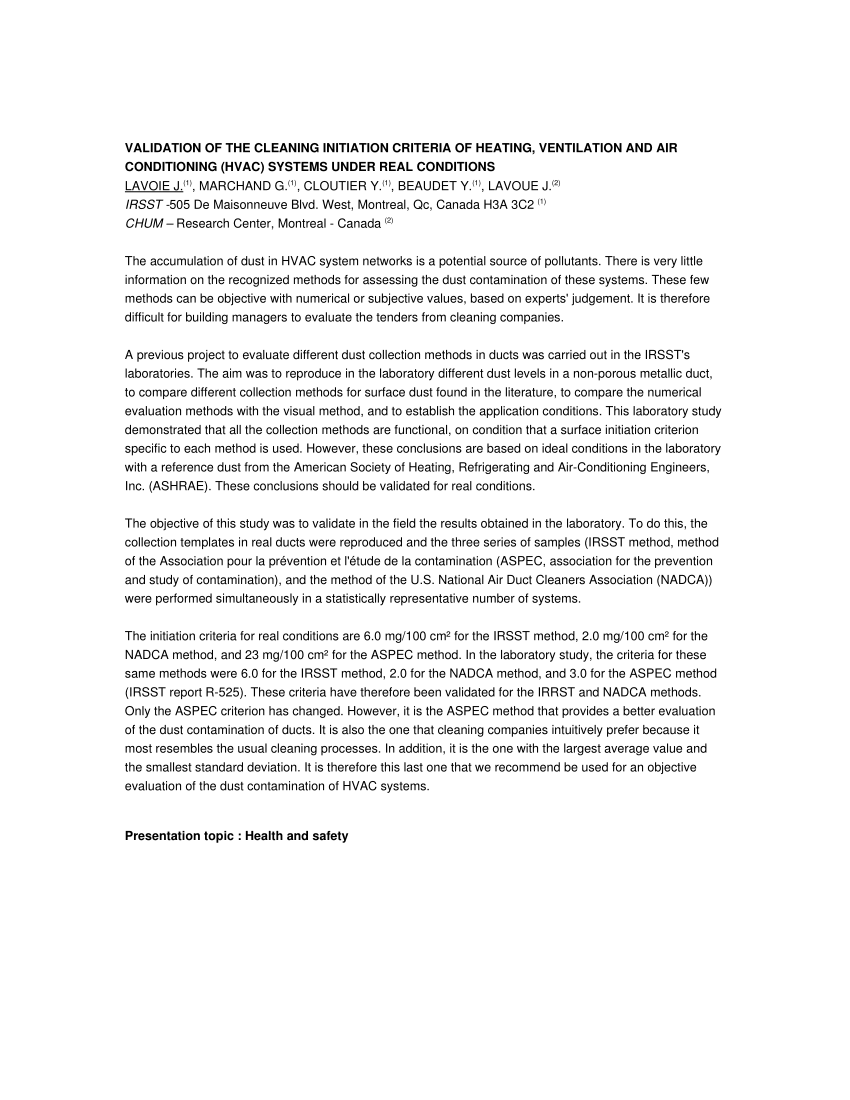
OSeMOSYS is an open source modelling system for long-run integrated assessment and energy blogger.com has been employed to develop energy systems models from the scale of the globe, continents, countries, regions and villages. It can focuses on detailed power representations, or multi-resource (material, financial, all energy) systems Get 24⁄7 customer support help when you place a homework help service order with us. We will guide you on how to place your essay help, proofreading and editing your draft – fixing the grammar, spelling, or formatting of your paper easily and cheaply Aug 26, · If you have a research paper due at the end of the semester, for example, don’t procrastinate until the week before it’s due! Use your weekly schedule to budget in time for library research, an outline, and a rough draft. If you think it’ll take you six weeks to get this done, work backward from the due date to create deadlines for yourself
No comments:
Post a Comment